Blog
Generative AI for Sustainability: Is It Possible?
Last updated: May, 2023
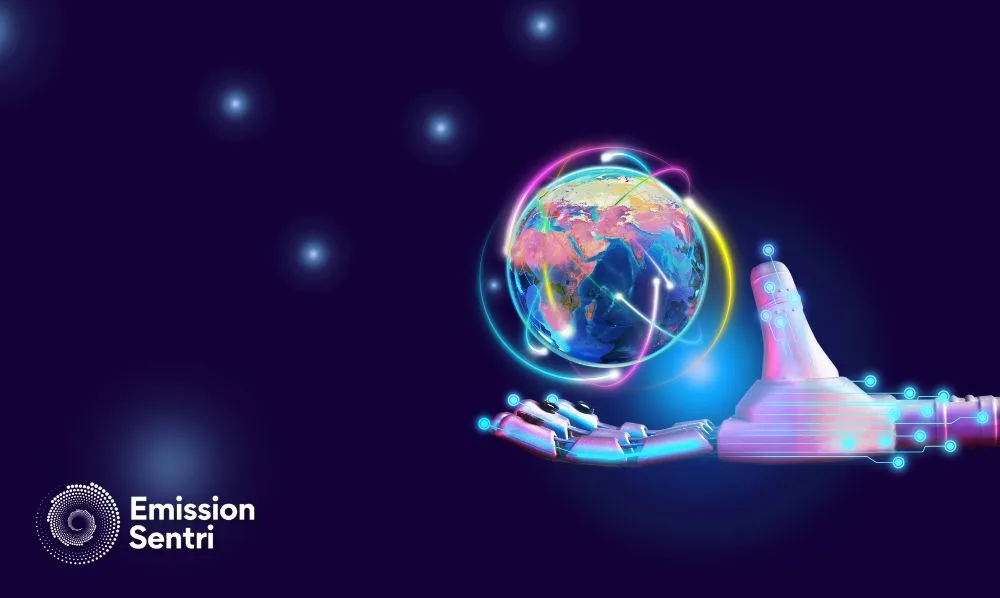
ChatGPT. DALL-E.
These are some of the most popular generative AI applications in the world.
While these tools are proving invaluable for productivity, they also have the potential for solving complex issues like global warming.
It can help drive ESG and net-zero goals across various industries by streamlining supply chains, adjusting energy needs in real time, or using predictive models for crop yields.
Yet, there is a downside.
The genius of AI’s efficiency comes with an unfortunate operating cost – high global greenhouse gas emissions.
This raises an important question: Is generative AI for sustainability even possible?
The Environmental Cost of Generative AI
While AI helps make our lives easier, it comes with a cost.
Data centers, crucial for AI’s operations, are responsible for 2–3% of global greenhouse gas (GHG) emissions. With the world’s data volume doubling every two years, this percentage will only keep increasing, further straining environmental resources.
Training advanced generative AI models is another concern regarding emissions. According to research, the recent class of GenAI models requires a ten to a hundred-fold increase in computing power to train models over the previous generation.
Not only does this exacerbate energy consumption, but it also significantly increases the carbon footprint associated with developing state-of-the-art AI technologies.
For instance, training a single large AI model like GPT-4 consumes about 300 tons of CO2. In comparison, the average person creates around 5 tons of CO2 a year.
The environmental cost of generative AI highlights the need to find a healthy balance between technological innovation and our planet’s health.
RELATED: How Can AI Create a Sustainable Future
The Paradox in Generative AI for Sustainability
Generative AI sits at the center of a sustainability paradox.
Its potential to solve critical environmental problems is undeniable, yet the process of developing such AI systems involves substantial energy consumption and CO2 emissions.
The paradox lies in the fact that while these technologies can optimize energy use, reduce waste, and enhance efficiency across industries, their training and operational phases exert considerable pressure on the environment.
With AI’s capability to drive progress toward climate change solutions, the balancing act requires a reevaluation of current practices and a shift towards more sustainable AI development methodologies, ensuring that the path to innovation aligns with the broader goals of environmental sustainability.
RELATED: How Can Artificial Intelligence Help in Climate Change?
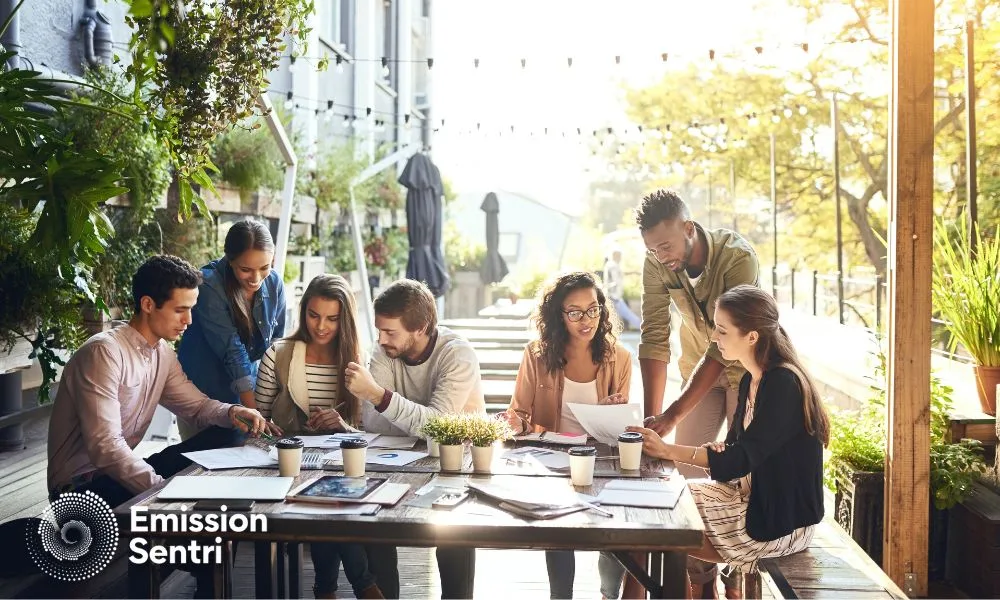
Pathways to Greener Generative AI for Sustainability
Innovative strategies are being explored to address the carbon footprint machine learning creates and minimize the environmental footprint of AI.
Algorithm Optimization
Algorithm optimization involves refining AI algorithms to perform tasks more efficiently, requiring less computational power and, consequently, less energy. This includes developing algorithms to achieve the same or better results with fewer iterations or less data processing.
By optimizing AI algorithms, it can lead to significant energy savings by using techniques like:
- Pruning (removing unnecessary neurons).
- Quantization (reducing the precision of the numbers used).
- Knowledge distillation (transferring knowledge from large models to smaller, more efficient ones).
Energy-Efficient Hardware
Energy-efficient hardware focuses on creating processors and computing infrastructures designed to handle AI workloads with minimal energy consumption.
It includes the use of specialized AI chips, such as Tensor Processing Units (TPUs) and Field-Programmable Gate Arrays (FPGAs), which can execute AI tasks more efficiently than general-purpose CPUs.
These technologies reduce the power required for AI computations, improve the overall performance of AI applications, and help make generative AI possible for sustainability.
Green Data Centers
Green data centers use renewable energy sources, like solar and wind, to power their operations, significantly reducing the carbon footprint associated with AI’s computational demands.
In addition to utilizing green energy, these data centers often incorporate advanced cooling technologies, energy-efficient servers, and other sustainable practices to minimize their environmental impact.
Corporate Responsibility and Future Directions
The momentum is building, with 61% of executives recognizing the importance of generative AI for their sustainability agenda and 69% planning to increase their investment in generative AI for sustainability.
However, integrating sustainability into AI development processes requires effort from all stakeholders.
Future directions must focus on translating this awareness into action by integrating green practices at every AI development and deployment stage.
Corporations have the potential to lead the charge in minimizing the environmental footprint of AI, setting new industry standards for sustainability.
AI for Environment: Wrapping Up
Generative AI for sustainability is possible and necessary. The environmental challenges posed by AI’s rapid development can be addressed through efforts focused on innovation and sustainability.
And Emission Sentri can help you track your progress toward corporate sustainability at scale. Connect all your emissions data into an Insights dashboard and receive smart recommendations on where your business and employees can reduce their carbon footprint.